Open platform for realizing zero defects in cyber-physical manufacturing
openZDM is an initiative that will develop and demonstrate in five representative production lines an open platform designed to realize Zero Defect Manufacturing.
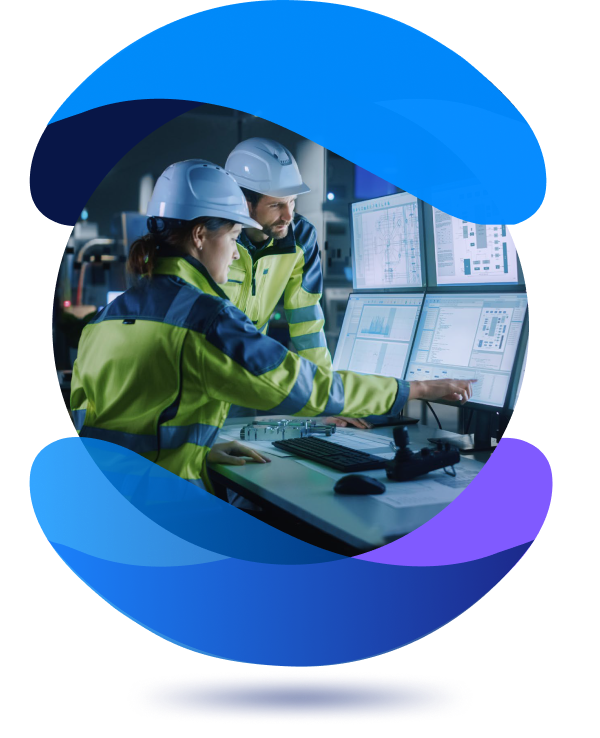
Welcome to the future of manufacturing excellence!
Our goal is to provide an innovative state-of-the-art integrated open platform that will combine advanced ICT solutions and innovative non-destructive testing, to support production networks’ zero-defect processes.
PILOTS
Deploy and demonstrate the openZDM solution
Trough five industrial pilots in real-life operational conditions, representing the largest part of the EU’s manufacturing sector, geographically, technologically and from their value chain positioning, we will test and finalise the technological tools and facilitate the adoption of the openZDM solution.
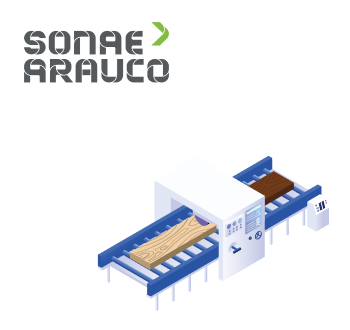
- Sonae Arauco
Decorative surfaced panels manufacturing demonstrator
The main goal of the pilot is the reduction of defects which will consequently [...]

- Aptiv
EV battery production demonstrator
The main goal of the pilot is to develop vision in VIS and IR [...]
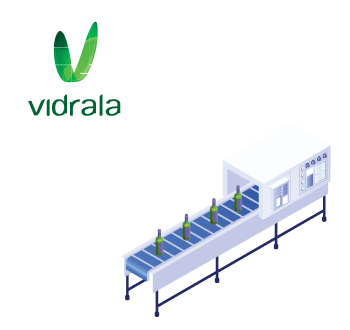
- Vidrala
Bottle manufacturing demonstrator
The main goal of the pilot is to provide a significant amount of raw [...]
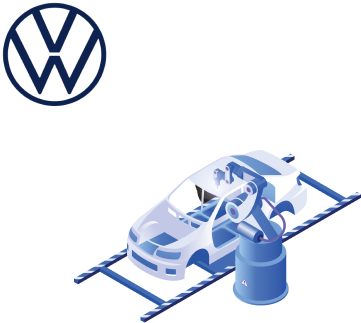
- VWAE
Vehicle body shop and final assembly demonstrator
The main goal of the pilot is the detection of the non-compliant products earlier [...]
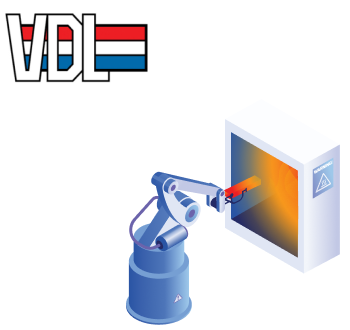
- VDLWEW
Trailing arm production process demonstrator
The main goal of the pilot is to adjust the production process to deviations [...]
INSIGHTS
Latest updates and news from the industry
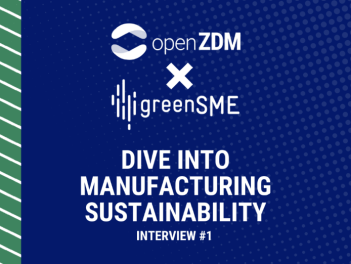
Dive into Manufacturing Sustainability with openZDM and GreenSME
We are excited to share that we started a set of interviews with the GreenSME project to address hot topics in sustainability…
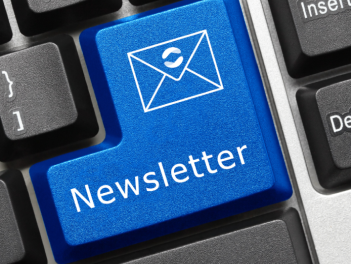
The fourth issue of the openZDM Newsletter is out now!
Don’t miss the chance to subscribe to the openZDM digital world and get the latest insights about manufacturing industry…
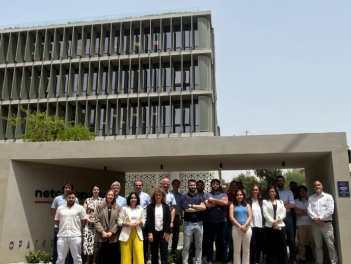
openZDM 5th General Assembly: Driving Zero-Defect Manufacturing
5th General Assembly Meeting of the openZDM project took place last May
22–23 in Athens…