PILOT CASE
Vidrala
Bottle manufacturing demonstrator
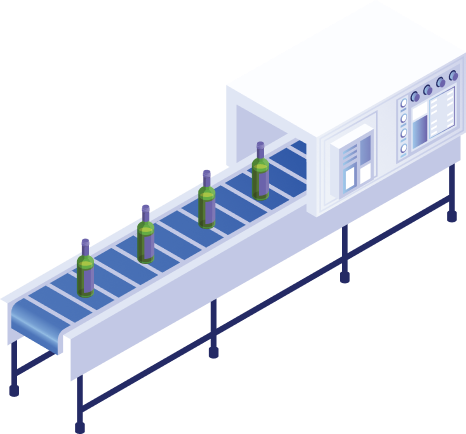
Pilot Description
Vidrala manufactures glass containers through a continuous process involving the melting of glass. This molten glass is brought through some forehearths to a spout, where some glass gobs are cut. These glass gobs will be formed into a bottle or jar each, without any addition or subtraction of mass. The conditions in which these gobs are formed depend on the temperature and homogeneity of the glass and will affect the regularity of the thickness of the bottle’s walls.
This relationship is not obvious. Some experience is held inside the factories, and some shapes are regarded as better than others, but this knowledge is qualitative and very difficult to optimise. In the first use case of Vidrala, the usage of modern data science tools should define the actual limits for the gob shape and the impacts of those limits.
Expected Goals
- Develop a gob shape control system and a thickness measurement predictor
Problem definition
Identified challenges
- The first challenge is the correct collection of data. The amount of suppliers and types of equipment involved requires a deep understanding and development of data tools.
- The second is defining the fine line that will make the balance between statistical significance, measurement errors, limits, and random variation.
Impact from openZDM technologies
The expected impact of openZDM project include reduction of defective products, early detection of problems and improvement on the quality levels. Besides, the involved technologies can help providing a better understanding of the manufacturing process itself.
The objective of the openZDM project is to work on the estimation of bottle wall thickness (WT) distribution using thermographic images at hot-end:
- Being able to use hot-end data to predict (create early warnings) wall thickness issues.
- Being able to optimize hot-end parameters (such as gob shape) to reduce the risk of having wall thickness issues.

Current status of the pilot:
- NDIs and their corresponding modifications to gather all images and related data are deployed in 2 pilot lines (CR10 and EL11).
- We are working on a predictive data-driven quality assessment module capable of predicting bottle thickness from infrared images.
- This model has been trained with a dataset generated using a unitary laser marking deployed in EL11 line, in order to provide one-to-one input-output data relations.
- Current preliminary results show good predictive capabilities for the short-term, but the performance of the model declines when using it with data a few days after the training period. This shows a need for a periodic retrain (or adjustment) of the model or trying a wider training dataset.
- Several dashboards have been developed within the DT solution.

